Challenges Automating the Law: AI in the Legal Sector
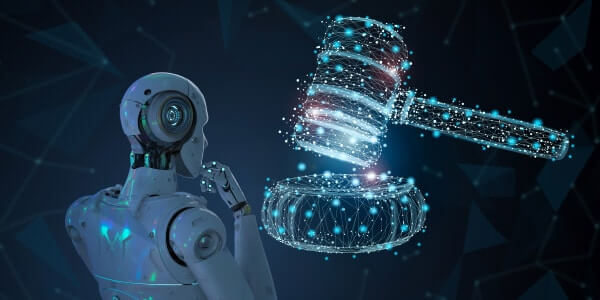
I. Introduction
Many lawyers debate whether technology should augment or outright replace humans in legal tasks. The difficulty with answering that question is that it is not clear what criteria should be used to determine which approach is preferable in a given application. In many cases it may be more cost effective or efficient to automate legal tasks, but doing so creates new issues that exceed the problems they were trying to solve in the first place. Therefore, this article will adopt a holistic cost/benefit analysis of whether legal automation is preferable to augmentation in various applications considering sociological, technical, and economic perspectives.
II. Applying AI to Law
When we think about automation, there are two distinct approaches to applying artificial intelligence (‘AI’) to legal practice. The older, rules-based systems deploy a ‘top-down’ approach to encode the decision criteria for a particular area (e.g., a flow chart determining liability in a case). The second approach, which has seen the most growth in recent years, uses statistical models or ‘machine learning’ (‘ML’). These systems use a ‘bottom-up’ approach starting with a large data set where a small subset is labelled according to an outcome you want to predict. The algorithm determines which variables in the training data are most correlated with that outcome (i.e., the best predictors). With sufficient computing power and data, ML systems can give “superhuman” results (e.g., analysing chest X-rays for early signs of lung cancer more accurately than humans).1
Recent advances in AI have led to sensational claims that legal services could be fully automated within decades. This has obvious appeal in that automation potentially improves accuracy (by removing human error), increases efficiency and productivity (given a machine’s superior computing power), and drastically reduce the costs of legal services. These could potentially help some of the issues with our current legal system such as ‘backlogged courts, overburdened public defenders, and swathes of defendants disproportionately accused of crimes’.2 However, now that the dust has settled and the legal profession has had a few years to start integrating AI tools, it has become clear that some areas legal practice are better suited for automation than others.
III. Challenges Automating the Law
a) Bespoke Work
One example of a legal process that many would agree has been successfully automated is ‘eDiscovery’, which uses ML models built on natural language processing (‘NLP’) to identify electronic material relevant to a dispute. Not only has the automation of this process resulted in significant costs savings (estimated to be up to 70% of total litigation costs), some studies have shown that the ‘results are significantly better than using human search.’3 Perhaps this does not come as a surprise when one considers the waning accuracy of a junior lawyer overworked and tired reviewing documents late into the night.
Similar legal tasks that have successfully incorporated AI technology include: contract analytics as part of M&A due diligence ‘based on a playbook of objectionable clause types’; using a chatbot for routine, low stakes legal advice like disputing a parking fine; drafting a standard legal document like a will using a digital app at the user’s convenience; searching vast legal databases with greater accuracy than humans; and making predictions about the outcome of litigation with >70% accuracy based on the facts of the case, prior precedent, and various other variables to inform settlement decisions.
The common factor in these use-cases is that they either involve analysis of large amounts of text-based data or the automation of repetitive, manual, standardised tasks typically performed by junior lawyers. It follows that the first major limitation to automation is legal work that is complex or unique that requires bespoke advice or nuanced work to be performed. Indeed, law firms using AI tools for due diligence have commented that they are ‘very well-suited to assisting the review of documents or contracts which are quite commoditised’, such as leases or supply agreements.4 The AI review is thus limited to identifying deviations from that standard form, leaving it to the lawyers to assess the legal implications / risks of those variations.5
Law firms must also consider the economics of implementing ML automation at scale. First, they need to obtain access to a sufficiently large, manipulable data set that is relevant for the intended use to train the systems on (not always available). Secondly, collecting and labelling data according to the variable of interest is expensive. This large upfront cost can likely be justified where the AI can be used for repeated work and its performance improved with supervised learning (e.g., Amazon’s ‘Data Flywheel’), but arguably it still makes economic sense for bespoke, one-off work to be completed by humans augmented with technology.
It may be the case that complex tasks can be broken down into many smaller tasks where automation can be implemented successfully. The legaltech firm Bryter cites the example of the manufacturing process for a car.6 There is no single machine that can build a car from scratch given it is a very complex process, but it can be effectively automated using several smaller machines along a conveyor belt that are specialised at individual components. The same logic may apply to automating legal processes where there are separate programmes to deal with each stage.
However, until such time that AI truly rivals the human mind, it is difficult to imagine machines replacing the many services that lawyers provide beyond strict legal advice. Lawyers are advocates for their clients, they provide innovative solutions to novel problems (with no prior examples of answers), and increasingly they partner with clients to provide commercial / strategic input. These services require creative and social intelligence, amongst other soft skills that are currently beyond the capabilities of AI technology, which remain better suited for pattern recognition and text-based work.7
With today’s technology, it is reasonable to conclude that some areas of law are more suited to automation than others: specifically, where there are ‘clearly defined rules and processes’, the logic and issues involved are relatively simple, the stakes are low, the work is repetitive and scalable, and there is minimal fact-finding.8
b) Vagueness
Having established that automation is ideal for areas of law where there are clearly defined rules and processes, the first obvious challenge to automating the law is its inherent vagueness. Both law and code attempt to provide for all possible outcomes to enable some activity to occur, but vagueness of meaning is a ‘pervasive feature of natural language’.9 Unlike Lex Informatica, legislation refers to nebulous standards like ‘reasonableness’ and ‘good character’ that inevitably turn on the evidence presented and require a value judgment (including the veracity of that evidence).
Timothy Endicott goes as far as arguing that vagueness is ‘both an important and unavoidable feature of law’.10 This is because the application of law involves a significant number of ‘borderline cases’ in which there is disagreement and doubt about how legal standards should be applied, so there needs to be discretion for judges to make decisions based on the facts of the case and past precedent. Nevertheless, this ambiguity – combined with the complexity of syntax and semantics – makes it very difficult to formalise law using axiomatic logic. For example, there are potentially an infinite number of behaviours that could be constituted as ‘reckless’ driving. The risk in attempting to codify all the possible scenarios is that you would inevitably miss some actions (or over-include arbitrary scenarios). It is therefore in society’s best interests to refer to a nebulous concept like recklessness (in addition to precise rules), that can be applied generally and developed through precedent to reflect changes in societal behaviours.
The DAO heist in 2016, where a user was able to exploit a loophole in the code to siphon off $60 million in Ethereum, is a classic example of a failure to predict all the potential actions that could be taken. In that case, the Ethereum community decided to ‘hard-fork’ the blockchain to restore funds to the original owners, thereby relying on an external concept of ‘fairness’ to remedy the situation despite the black-and-white coding.11 Consequently, many academics believe the DAO is a prime example of why full automation will not work and that we should instead be wrapping smart contracts with traditional legal contracts (law trumping code). If a system does not produce the results the parties expected, there is need for a superior set of rules that apply to it. In a similar vein, equity law is a branch of the legal system based on principles of fairness and justice that has developed to fill the gaps of statute, recognising that the concept of law is greater than the words written on a piece of paper.
The DAO also demonstrates that automation of legal practice introduces the risk of new technical forms of failure. For example, cyber criminals may engage in ‘spoofing’ where they disguise communications as coming from a trusted, known source to obtain personal information, distribute malware or conduct denial-of-service attacks. Similarly, someone could potentially fool a NLP system by changing key words to try and get the desired outcome. Deep neural networks are also vulnerable to adversarial perturbations.12 These vulnerabilities add weight to the idea of needing recourse to more flexible legal principles to override code where appropriate. Other significant costs of automation include secure storage of huge amounts of data and the environmental impact of blockchain mining.
Modern jurisprudence requires that lawyers and judges apply a contextual and purposive approach to interpreting legislation. In other words, one should consider the economic and moral underpinning of the law – the ‘spirit of the law’ and what it was intended to achieve – rather than being constrained to the dictionary definition of the words used. The ‘always speaking’ principle advocated by Lord Burrows QC suggests that ‘[a] statute may apply to circumstances which could not possibly have been foreseen at the time the statute was passed’.13 Hence, the law needs to be construed in the context of changes to the natural world, technology, and social attitudes.
There will inevitably be rare, unpredictable ‘edge’ cases that an automated system does not know how to handle because it has not seen it before. Further, the law was not designed to be static and AI systems would need to be reprogrammed / retrained to reflect new interpretations of / changes to law. Thus, automating the law is neither practical nor desirable where vagueness is embedded in the rules.
c) The Human Elementk
Another example demonstrating the limitations of legal automation is the emergence of online dispute resolution, which has accelerated significantly during the COVID-19 pandemic. Tom Tyler argues that participants in the justice system not only care about the outcome of dispute resolution but ‘their feelings and behaviours have an important ethical and moral component’.14 Thus, we should not be focused solely on whether an automated system produces the same, or even better, results than humans – we should also consider the parties’ desire to have their day in court and be judged by their peers. For some people, the ritualistic aspects of the courtroom and having a case resolved by human beings are important for their sense of justice.
There are also concerns about ‘digital exclusion’ of disadvantaged and vulnerable people from online justice services who struggle to access or use technology, particularly when considering the human rights of people with disabilities.15 There is therefore a question of whether automation will enhance access to justice or reduce it for certain demographics. That being said, ‘the general trend is towards increasing digital capability’ and ‘ever-more user-friendly technologies’. Furthermore, investment to improve digital skills and access to the minority of people at risk may be justified in light of the cost-savings that automation can produce overall. There is also a movement to designing systems centred on the user with participative approaches that may mitigate these concerns.16 Therefore, it would be rash to say that augmentation is always preferable in a dispute resolution context, particularly where the stakes are low (e.g., Amazon vendor disputes), but more needs to be done to reduce the barriers of participation in digital justice.
d) Bias & Accuracy
One step further than online dispute resolution, legal experts are currently grappling with where to draw the line for full automation vs decision support in dispute resolution (although this line may blur to the extent human decision-makers systematically over-rely on computer output). For example, a recent study looked at automating SME dispute resolution to ameliorate the issue of late payments.17
Putting aside the issue discussed above that codifying the law is extremely difficult in some cases due to its necessary vagueness, another issue that arises from automated dispute resolution (‘ADR’) is that it may amplify existing biases and prejudice that are reflected in the input data. Joy Buolamwini highlighted an alarming example of gender and racial bias in technology when proving that facial recognition technology incorrectly recognised women with darker skins tones as men because there was not sufficient representation of minorities in the data set.18 Secondly, the systems themselves may be biased if it is based on correlation and not causation (e.g., COMPAS demonstrating ‘racial bias and inaccuracy by incorrectly associating higher risk of reoffending with black people’). Of course, humans do not always make perfectly accurate, unbiased decisions, but the risk is heightened with ADR due to the scalability of its application and may in fact increase litigation.
e) Transparency
One could argue automated systems are more transparent than humans – especially rules-based systems that are constrained to the input data and the algorithm that processes it, but even ML systems involve the selection and labelling of training data that can be scrutinised. ‘One of the greatest promises of ADR is its presumed ability to eliminate subconscious bias that inevitably underlies all human decision making.’ Concerns about ML black box systems are also likely overblown given it is possible to use interpretable white-box systems and techniques are being developed to make them more explainable.
Hence, the bias issues of ADR can be mitigated with rigorous scrutiny in building the ADR models and fair data selection. For example, some algorithms have already been trained to include ‘anti-discriminatory constraints during data processing or removing the sources of bias prior to processing.’19 Alternatively, AI could be limited to appraising human reasoning – confirming whether they made correct, consistent, and predictable results. AI could even be a tool to identify bias like the hungry judge effect.
IV. Conclusion
It would not be correct to say that augmentation is always preferable to automation because we have shown above that there have been very successful examples of technology automating repetitive, time-consuming, and standardized tasks that machines thrive on; thereby lowering legal costs and allowing lawyers to spend more time on high-value tasks. However, there remain several challenges to automating legal practice that make augmentation preferable in many cases including the vagueness of law, the need for purposive interpretation and discretion in individual cases, bias and accuracy concerns, and the human element of justice.
It may be possible that with developments in technology like General AI, ML programs could be taught to interpret even nebulous concepts like reasonableness at a suitable level of accuracy based on a very large data set. But for now, it is appropriate to take a cost/benefits view, considering wider societal and ethical implications, and recognise that some areas of law are more suited to automation than others.
Commentators including Richard Susskind argue we should be taking a pragmatic approach to automation appreciating that although it may not be perfect, the overall efficiency gains may outweigh the loss of discretion in individual cases. In other words, for some people automated solutions may be better than nothing and a certain level of tolerance to inaccuracy is appropriate. For example, the UK government has said the automation of universal credit helped ‘it cope with an unprecedented surge in the number of people claiming Universal Credit’ during COVID-19 lockdowns.20 Likewise, the time-saving of automated contract review may outweigh a certain level of decreased accuracy.
Overall, our goal should not be to fully automate legal processes, but instead to improve efficiency incrementally, however possible. Partial automation, with appropriate human oversight and legal framework, would allow us to capture the best of both the human and machine worlds: replacing humans in some tasks while augmenting the capabilities of human lawyers in other tasks.
Footnotes
[1] John Armour, Richard Parnham, Mari Sako ‘Augmented Lawyering’ (2020) ECGI, 16. [2] bryter.com/decision-automation/what-is-the-status-quo-automation-in-law [3] John Armour, ‘Automating the Law’ (2021) Law & Computer Science Seminar 6 slides [4] www.imprima.com/blog/expert-panel-how-ai-is-changing-legal-due-diligence [5] ibid [6] Bryter (n 2) [7] Armour (n 1) 18 [8] https://bryter.com/blog/legal-automation-status-quo/?utm_source=google&utm_medium=organic [9] Geert Keil and Ralf Poscher, ‘Vagueness and Law, Philosophical and Legal Perspectives’ (2016) Oxford University Press, 1. [10] Timothy Endicott, ‘Law Is Necessarily Vague’ (2001) Legal Theory, 379. [11] https://www.coindesk.com/learn/2016/06/25/understanding-the-dao-attack/ [12] https://www.bosch-ai.com/research/research-applications/universal-adversarial-perturbations/ [13] academic.oup.com/slr/advance-article/doi/10.1093/slr/hmaa019/5924582#330287680 [14] Tom Tyler, ‘Procedural Justice’ in The Blackwell Companion to Law and Society (2004), 449. [15] Justice Committee, ‘Preventing Digital Exclusion from Online Justice’ (2018). [16] Patrizia Marti and Liam Bannon, ‘Exploring User-Centred Design in Practice: Some Caveats’ (2009). [17] ‘Feasibility study for an online dispute resolution platform for SMEs’ (2021) LawtechUK. [18] Malwina Wojcik, ‘Machine-Learnt Bias? Algorithmic Decision Making and Access to Criminal Justice’ (2020) Legal Information Management, 99. [19] ibid 100 [20] www.hrw.org/report/2020/09/29/automated-hardship/how-tech-driven-overhaul-uks-social-security-system-worsens